Acceleration of aerosol product retrieval algorithm for Earth observation satellite using Artificial Intelligence (AI)
JAXA Supercomputer System Annual Report April 2019-March 2020
Report Number: R19EDR20100
Subject Category: Space Technology
- Responsible Representative: Makiko Hashimoto, Space Technology Directorate I, Earth observation research center
- Contact Information: Makiko Hashimoto(hashimoto.makiko@jaxa.jp)
- Members: Makiko Hashimoto, Chong Shi, Kazuya Muranaga, Takashi Nagao
Abstract
With the advancement of satellite sensors, it is required to improve the accuracy of aerosol product estimation and estimate the multiple products simultaneously. Under such circumstances, the conventional LUT method and the method using direct radiative transfer require a huge amount of data and computation time, and it is impractical to extend the algorithm using those method. Therefore, we accelerate the algorithm by an Artificial Intelligence (AI) technique (here, a neural network technique). By using a supercomputer, it is possible to create a large amount of taining data in a short period of time to accelerate an algorithm (radiative transfer model) by using AI techniques. Applying the created general-purpose accelerated radiative transfer solver to GOSAT-2 / CAI-2, Himawari-8 / AHI, GOSAT / CAI, GCOM-C / SGLI, we aim to respond to the increase in data due to the higher resolution of atmospheric observation satellites and aim to provide near real-time data.
Reference URL
N/A
Reasons and benefits of using JAXA Supercomputer System
Importance of using JSS2: To educate radiative transfer model required for satellite analysis, it takes about 1.2 billion radiative transfer calculations to create training data. By using a large number of node (600 nodes, 32 cores/node) in JSS2 SORA-MA that we can use, the calculations that take 11 years by 1 node can be performed in about 10 days. In other words, the use of SORA-MA makes it possible to accelerate satellite data analysis. The accelerated radiative transfer model (called NN solver) educated using the calculated training data is applied to the CAI-2 Level 2 aerosol characteristic product derivation algorithm launched in 2018, and to the already launched Himawari and GCOM-C data. If necessary, the NN solver will be improved. As described above, it is necessary to create training data in a short time, and it is very important to use JSS2 SORA-MA.
Achievements of the Year
In this year, we improved the algorithm and the calculation time for deriving aerosol properties for the GOSAT-2/CAI-2 Level2. The acceleration has made it possible to analyze one day of observation data in one day.
-The JAXA supercomputer was used to create training data to accelerate the radiative transfer calculations used by the aerosol retrieval algorithm. The acceleration method is a neural network method (Takenaka +, 2019.NN + Active Learning). The number of training data is 1.2 billion ( 600 million in ocean case and 600 million in land case), and the time required for calculation is 9 days in ocean case and 7 days in land case. Compared to the original radiative transfer model, accuracy is less than 5.0E-4, calculation speed is reduced to ~ 0.45sec/time/wavelength (land) and ~1sec/time/wavelength (ocean) to 0.45E-6sec/time/wavelength (Fig. 1) (Takenaka, EORC).
– In addition to aerosol characteristics, we are developing an aerosol derivation algorithm that combines ocean properties such as chlorophyll, CDOM, and Sediment (SS).100 million training data for ocean case are calculated using JAXA supercomputer. Achieving a speed of more than 50,000 times with the AI-accelerated radiative transfer module(Fig. 2). Applying the algorithm for GOSAT-2/CAI-2, we derived the aerosol characteristics of the global ocean (Fig. 3) (Shi, EORC).
– Using the training data set calculated by JAXA supercomputer, we also have created this high-speed module for Himawari and GCOM-C (Takenaka, EORC) and applied it to data analysis of GCOM-C / SGLI for research (Sekiguchi, Tokyo University of Marine Science and Technology).
– As described above, as a result, we were able to create an AI-accelerated radiation transfer module and apply it to a satellite aerosol analysis algorithm.
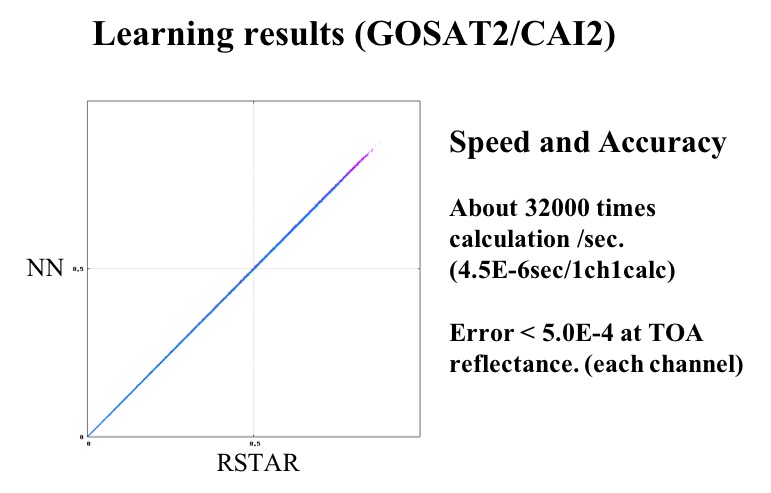
Fig.1: Learning results for GOSAT-2/CAI-2. Speed and Accuracy. Comparison between the original radiative transfer model and the acceleratied modile (Neural Network solver). X-axis is original radiative transfer model, RSTAR, results, and Y-axis is the learning result.
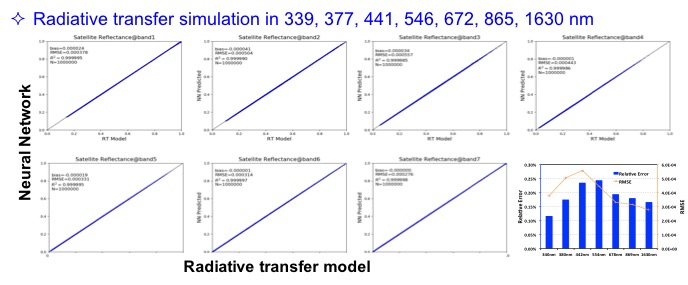
Fig.2: Comparison between the original radiative transfer model and the acceleratied modile including ocean properites. Based on the training data from the JSS2, an accelerated module (Neural Network solver) can be developed to replace the radiative transfer model during the satellite retrieval. X-axis is original radiative transfer model, RSTAR, results, and Y-axis is the learning result.
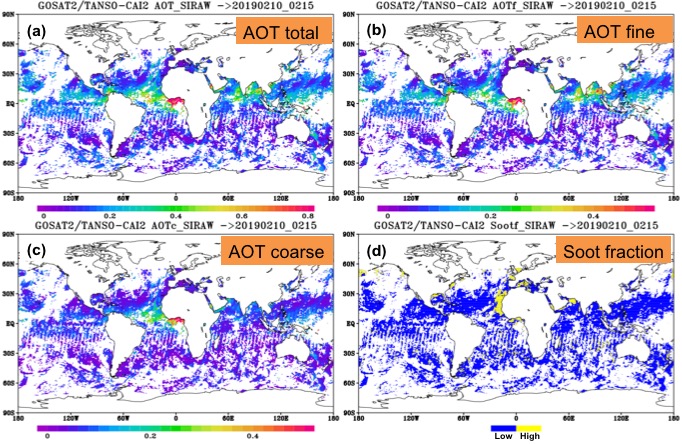
Fig.3: Application of aerosol retrieval. Global aerosol distribution, total aerosol optical thickness (AOT), fine AOT, coarse AOT and soot volume fraction, by GOSAT2/TANSO-CAI-2 from February 10 to 15 in 2019.
Publications
– Peer-reviewed papers
1) Shi, C., M. Hashimoto and T. Nakajima, 2019: Remote sensing of aerosol properties from multi-wavelength and multi-pixel information over the ocean. Atmos. Chem. Phys., 19, 2416-2475.
2) Ma, R., H. Letu*, K. Yang, T. Wang, C. Shi, J. Xu, J. Shi, C. Shi, and L. Chen (2020), Estimation of Surface Shortwave Radiation from Himawari-8 Satellite Data Based on a Combination of Radiative Transfer and Deep Neural Network, IEEE transactions on geoscience and remote sensing, doi:10.1109/TGRS.2019.2963262.
3) Peng, Z., H. Letu*, T. Wang, C. Shi*, C. Zhao, G. Tana, N. Zhao, T. Dai, R. Tang, and H. Shang (2019), Estimation of shortwave solar radiation using the artificial neural network from Himawari-8 satellite imagery over China, Journal of Quantitative Spectroscopy and Radiative Transfer, 106672.
– Oral Presentations
1) Shi C., Hashimoto M., Takenaka H., Nakajima T., Retrieval of Aerosol Optical Properties Using GOSAT/ TANSO-CAI and GOSAT2/TANSO-CAI2, The 15th International Workshop on Greenhouse Gas Measurements from Space (IWGGMS-15), 3-5 June, 2019, Hokkaido, Japan.
2) Hashimoto M., Chong S., Yoshida M., Kikuchi M., M. Nagao T., and Nakajima T., Monitoring of Air Pollution from Space by GOSAT, GOSAT-2 and Himawari-8. LCLUC SARI 2019, 23 July, 2019, Johor Bahru, Malaysia.
– Poster Presentations
1) Shi C., Nakajima T., Hashimoto M., Takenaka H., Simultaneous retrieval of aerosol optical properties and ocean color based on the optimal estimation approach, European Geosciences Union, 7-12 April, 2019, Vienna, Austria.
2) Shi C., Nakajima T., Hashimoto M., Takenaka H., Remote sensing of aerosol optical properties and water-leaving radiance based on the optimal estimation approach, Japan Geoscience Union Meeting, 26-30 May, 2019, Chiba, Japan.
3) Hashimoto M., Takenaka H., Nakajima T. and Higurashi A., Aerosol retrieval and PM2.5 from space using GOSAT/TANSO-CAI, Japan Geoscience Union Meeting, 30 May, 2019, Chiba, Japan.
4) Hashimoto M., Takenaka H., Higurashi A. and Nakajima T., GOSAT-2/TANSO-CAI-2 and the aerosol product. IWGGMS, 4 June, 2019, Hokkaido, Japan.
5) Hashimoto M., Chong S., Takenaka H. and Nakajima T., Comparison of aerosol properties from GOSAT-2/TANSO-CAI-2 with ground-based observation and the error analysis using aerosol retrieval algorithm, JMA fall meeting 2019, 30 October, 2019, Fukuoka, Japan.
6) Hashimoto M., Takenaka H., Chong S. and Nakajima T., GOSAT-2/TANSO-CAI-2 Aerosol properties by Multiple wavelengths and pixels method, AGU Fall Meeting 2019, 9 December, 2019, San Francisco, USA.
Usage of JSS2
Computational Information
- Process Parallelization Methods: N/A
- Thread Parallelization Methods: A large amount of computation was performed using the number of available cores in MA
- Number of Processes: 1
- Elapsed Time per Case: 15 Minute(s)
Resources Used
Fraction of Usage in Total Resources*1(%): 1.39
Details
Please refer to System Configuration of JSS2 for the system configuration and major specifications of JSS2.
System Name | Amount of Core Time(core x hours) | Fraction of Usage*2(%) |
---|---|---|
SORA-MA | 12,660,282.73 | 1.54 |
SORA-PP | 0.00 | 0.00 |
SORA-LM | 0.00 | 0.00 |
SORA-TPP | 0.00 | 0.00 |
File System Name | Storage Assigned(GiB) | Fraction of Usage*2(%) |
---|---|---|
/home | 25.43 | 0.02 |
/data | 10,274.26 | 0.18 |
/ltmp | 5,208.34 | 0.44 |
Archiver Name | Storage Used(TiB) | Fraction of Usage*2(%) |
---|---|---|
J-SPACE | 0.00 | 0.00 |
*1: Fraction of Usage in Total Resources: Weighted average of three resource types (Computing, File System, and Archiver).
*2: Fraction of Usage:Percentage of usage relative to each resource used in one year.
JAXA Supercomputer System Annual Report April 2019-March 2020