Research for data assimilation of satellite global rainfall map
JAXA Supercomputer System Annual Report February 2022-January 2023
Report Number: R22ER0201
Subject Category: Space Technology
- Responsible Representative: Riko Oki, Director, Space technology Directorate I, Earth Observation Research Center
- Contact Information: Space technology Directorate I, Earth Observation Research Center, Takuji Kubota(kubota.takuji@jaxa.jp)
- Members: Koji Terasaki, Yingwen Chen, Kaya Kanemaru, Shunji Kotsuki, Hisashi Yashiro
Abstract
This study explores an effective use of satellite data including GSMaP and GPM/DPR through an advanced ensemble data assimilation method for improving numerical weather prediction (NWP) and pioneering a new precipitation product based on an NWP model and satellite observations, named as NICAM-LETKF JAXA Research Analysis (NEXRA).
Reference URL
Please refer to ‘JAXA Realtime weather watch‘.
Reasons and benefits of using JAXA Supercomputer System
In this study, the JSS3 is used for the NICAM-LETKF experiments to assimilate satellite observations and to conduct NWP model forecasts. The JSS3 is an essential infrastructure for our study to conduct massive computations for the ensemble-based data assimilation and ensemble atmospheric simulations.
Achievements of the Year
(1) Data assimilation of GPM DPR radar reflectivity for cloud microphysics parameter estimation
Using the NICAM-LETKF system and GPM DPR observations, this study estimates a model cloud physics parameter corresponding to snowfall terminal velocity. To overcome the difficulties of long revisiting intervals in GPM DPR and precipitation location errors, we proposed a method to estimate a parameter of snowfall terminal velocity using GPM DPR. The parameter estimation effectively mitigated the gap between simulated and observed radar reflectivity, resulting in improvement in 6-hour precipitation forecasts (Fig. 1) and outgoing longwave and shortwave radiation biases (Fig. 2).
(2) Development of the next-generation NEXRA
We have developed a new NEXRA system this fiscal year by modifying the NICAM-LETKF code. This new NEXRA system was developed based on the NICAM-LETKF system with horizontal resolution of 56 km (GL07). Currently, this system is under the spin-up process with 64 ensemble size and will be extended to 128 ensemble size after checking the system performance. We have confirmed that the precipitation pattern is well reproduced in the new NEXRA (Fig. 3).
(3) Ensemble typhoon track forecasts by the NEXRA
In this fiscal year, we have routinely operated 7-day ensemble forecasts with 5 members once a day on a trial basis. We evaluate the 7-day forecast results at the initial time from 2022.08.31 to 2022.09.03 00UTC by comparing the best track of typhoon HINNAMNOR from Japan Meteorological Agency (JMA) (Fig. 4). All forecasts reproduce the northward passages of HINNARMNOR even at the early stage. Over time, the uncertainty of the typhoon track in the ensemble forecasts is reduced, and the ensemble forecasts are closer to the actual typhoon track.
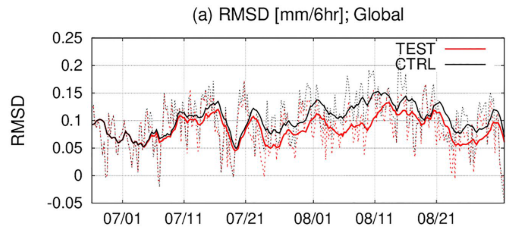
Fig.1: Time series of 6 hour forecast precipitation verifications relative to GSMaP NRT data for (a) root mean square difference (RMSD) for TEST (red) and CTRL (black) experiments with and without the parameter estimation, respectively. Dashed lines indicate 6-hourly forecasts; solid lines indicate the 3-day running mean. The abscissa is the validation month/date in 2014.
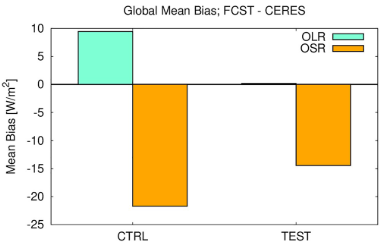
Fig.2: Global mean radiation bias (Unit: W m^-2) relative to the CERES data for the TEST and CTRL experiments with and without the parameter estimation, respectively, averaged over 1-31 August 2014. Cyan and orange bars indicate biases for outgoing longwave radiation (OLR) and outgoing shortwave radiation (OSR), respectively.
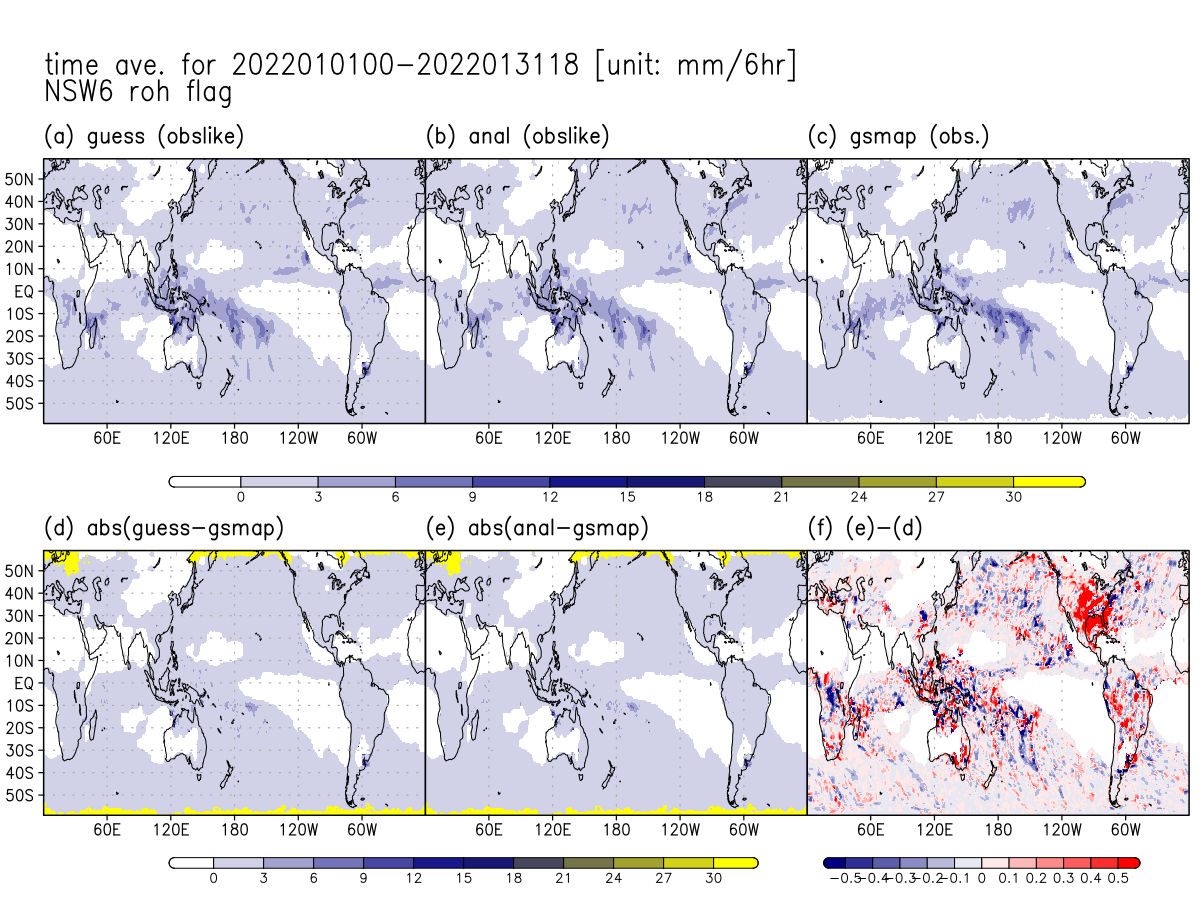
Fig.3: Monthly mean precipitation in January 2022 from (a) forecasts and (b) analyses in the new NEXRA, and (c) GSMaP. The absolute difference between (c) and (a) in (d), (b) and (a) in (e). (f) The difference between (e) and (d). In (f), the cold (warm) color indicates the improvement (degradation) by analyses. Unit is mm/6hr.

Fig.4: Typhoon HINNAMNOR track from 7-day ensemble forecasts with 5 member from 00UTC (09JST) on (a) 31st August, (b) 1st, (c) 2nd, and (d) 3rd September 2022. Gray lines indicate the ensemble mean, other lines denote each member, and black dots show the best track from the JMA.
Publications
– Peer-reviewed papers
Terasaki, K., and T. Miyoshi, 2022: A 1024-Member NICAM-LETKF Experiment for the July 2020 Heavy Rainfall Event, SOLA 2022 Volume 18A Issue Special_Edition Pages 8-14, doi:10.2151/sola.18A-002
Kotsuki, S., Miyoshi, T., Kondo K., and Potthast R. 2022: A Local Particle Filter and Its Gaussian Mixture Extension Implemented with Minor Modifications to the LETKF. Geosci. Model Dev., 15, 8325-8348, doi:10.5194/gmd-2022-69
Ohishi, S., T. Hihara, H. Aiki, J. Ishizaka, Y. Miyazawa, M. Kachi, and T. Miyoshi, 2022: An ensemble Kalman filter system with the Stony Brook Parallel Ocean Model v1.0, Geosci. Model Dev., 15, 8395-8410, doi: 10.5194/gmd-15-8395-2022
LIANG Jianyu, TERASAKI Koji, MIYOSHI Takemasa, A Machine Learning Approach to the Observation Operator for Satellite Radiance Data Assimilation, JMSJ, 101, 79-95, https://doi.org/10.2151/jmsj.2023-005
Ohishi, S., Miyoshi, T., and Kachi, M.: An ensemble Kalman filter-based ocean data assimilation system improved by adaptive observation error inflation (AOEI), Geosci. Model Dev., 15, 9057-9073, https://doi.org/10.5194/gmd-15-9057-2022
Momoi, M., Kotsuki, S., Kikuchi, R., Watanabe, S., Yamada, M., and Abe, S. (2023): Emulating rainfall-runoff-inundation model using deep neural network with dimensionality reduction. Artificial Intelligence for the Earth Systems, 2, 1-25, doi:10.1175/AIES-D-22-0036.1
Kotsuki, S., Terasaki, K., Satoh, M., & Miyoshi, T. (2023). Ensemble-based Data Assimilation of GPM DPR Reflectivity: Cloud Microphysics Parameter Estimation with the Nonhydrostatic Icosahedral Atmospheric Model (NICAM). Journal of Geophysical Research: Atmospheres, 128, e2022JD037447, https://doi.org/10.1029/2022JD037447 (in press)
Ohishi, S., T. Miyoshi, and M. Kachi: LORA: A local ensemble transform Kalman filter-based ocean research analysis, Ocean Dynamics, in press
– Invited Presentations
2022/5/31 Takemasa Miyoshi. Data assimilation research using Fugaku at RIKEN, Meteorology Colloquium, LMU Munich
2022/6/9 Takemasa Miyoshi, A. Amemiya, T., Honda, T., Otsuka, S., Maejima, Y., Taylor, J., Tomita, H., Nishizawa, S., Sueki, K.Yamaura, T., Ishikawa, Y., Satoh, S., Ushio, T., Koike, K., Hoshi, E., Big data assimilation: Real-time 30-s-update forecast experiments using Fugaku in Tokyo in 2021, ISDA2022, Fort Collins, Colorado, Keynote
2022/6/20 Takemasa Miyoshi, Fusing Big Data and Big Computation in Numerical Weather Prediction, International HPC Summer School 2022, Athens, Greece, Keynote
2022/8/5 Takemasa MIYOSHI, Arata AMEMIYA, Takumi HONDA, Shigenori OTSUKA, Yasumitsu MAEJIMA, James TAYLOR, Hirofumi TOMITA, Seiya NISHIZAWA, Kenta SUEKI, Tsuyoshi YAMAURA, Yutaka ISHIKAWA, Shinsuke SATOH, Tomoo USHIO, Kana KOIKE, Erika HOSHI, Big Data Assimilation: Real-time 30-s-update Forecast Experiments Using Fugaku in Tokyo in 2021, AOGS2022 Annual Meeting, Online
2022/8/24 Takemasa Miyoshi, Big Data Assimilation Real-Time 30-s-update Experiments Using Fugakuin Tokyo in 2021, WWRP Symposium, Online
2022/9/6 Takemasa Miyoshi, Big Data Assimilation Revolutionizing Numerical Weather Prediction Using Fugaku, 2nd US-Japan Workshop on Data-Driven Fluid Dynamics, Kobe, Keynote
2022/11/15 Takemasa Miyoshi, Big Data Assimilation: Real-time 30-s-update Torrential Rain Forecast Using Fugaku in Tokyo in 2021, The 5th ISEE Symposium Toward the Future of Space-Earth Environmental Research, Nagoya
2022/11/23 Takemasa Miyoshi, Big Data Assimilation Revolutionizing Numerical Weather Prediction Using Fugaku, University of Reading Data Assimilation Research Center and RIKEN Online Joint Seminar Series, Reading, UK
2022/11/24 Takemasa Miyoshi, Big Data Assimilation revolutionizing numerical weather prediction using Fugaku, Statistical Science Seminar, University of College London, UK
2022/12/1 Takemasa Miyoshi, Big Data Assimilation Revolutionizing Numerical Weather Prediction Using Fugaku, AOSC Seminar, University of Maryland, College Park, US
2023/1/28 Konduru Rakesh Teja, How to make high resolution simulations representative of future climate?, Examining the impact of Aerosol, Urbanization, and Irrigation on extreme rainfall occurrences over India using Cloud-Resolving Simulations, Indian Institute of Technology Madras, Online
2023/2/3 Takemasa Miyoshi, Big Data Assimilation Revolutionizing Numerical Weather Prediction Using Fugaku, DA Forum by University of Melbourne, Melbourne, Australia
– Oral Presentations
2022/5/23 Ting-Chi Wu, Koji Terasaki, Shunji Kotsuki, and Takemasa Miyoshi, Examining the Sensitivity of the Accuracy of EFSO to Ensemble Size, EGU 2022 General Assembly, Vienna, Austria (hybrid)
2022/5/23 Takemasa Miyoshi, Big Data Assimilation: Real-time 30-s-update Forecast Experiments Using Fugaku in Tokyo in 2021, JpGU Meeting 2022, Chiba (hybrid)
2022/5/23 Takemasa Miyoshi, Advances and applications of satellite data assimilation of clouds, precipitation, and the ocean, JpGU Meeting 2022, Chiba (hybrid)
2022/5/24 Takemasa Miyoshi, Experimental platform for design and advance evaluation of frequent satellite observations to innovate weather, ocean and land surface predictions, JpGU Meeting 2022, Chiba (hybrid)
2022/5/26 Kotsuki, S., and Bishop, C., A Multi-scale Localization for the Local Ensemble Transform Kalman Filter with Attenuation of Ensemble Perturbation, JpGU Meeting 2022, Chiba (hybrid)
2022/5/26 Ohishi, Shun, Takemasa Miyoshi, and Misako Kachi, LETKF-based Ocean Research Analysis (LORA) in the Western North Pacific region, JpGU Meeting 2022, Chiba (hybrid)
2022/8/5 Jianyu Liang, Norihiko Sugimoto, and Takemasa Miyoshi, A Machine Learning Approach To The Observation Operator For Satellite Radiance Data Assimilation, AOGS2022 Annual Meeting, Online
2022/8/5 Shun Ohishi, Takemasa Miyoshi, and Misako Kachi, LETKF-based Ocean Research Analysis (LORA) in the Western North Pacific region, AOGS2022 Annual Meeting, Online
2022/9/26 Takemasa Miyoshi, RIKEN’s activities on fusing AI and data assimilation in numerical weather prediction, Sea tech week 2022, Brest, France
2022/10/7 Shun Ohishi, Takemasa Miyoshi, and Misako Kachi, LETKF-based Ocean Research Analysis (LORA) in the Western North Pacific region, ISDA-online, Online
2022/10/26 Rakesh Teja KONDURU, Jianyu Liang, Koji TERASAKI, and Takemasa MIYOSHI: Estimating optimal vertical localization in assimilating AMSU-A satellite radiances at different frequencies in the NICAM-LETKF OSSE framework, MSJ Autumn, Sapporo
– Poster Presentations
2022/8/3 Ying-Wen Chen, Koji Terasaki, Shunji Kotsuki, Kaya Kanemaru, Masaki Satoh, Takemasa Miyoshi, and Takuji Kubota: The development of a weather information system based on the NICAM-LETKF assimilation system, AOGS2022 Annual Meeting, Online
2022/11/15 Ohishi, Shun, Takemasa Miyoshi, and Misako Kachi, LETKF-based Ocean Research Analysis (LORA): Assimilating high-frequency satellite observations, Joint Workshop of the OS-Eval TT and CP-TT and SynObs Kick-Off, Tsukuba
2022/12/13 Takemasa Miyoshi, Shigenori Otsuka, Arata Amemiya, Jianyu Liang, Fusing AI and data assimilation with HPC in numerical weather prediction, AGU Fall meeting (AGU22) , Chicago, US
2023/2/6 Ohishi, Shun, Takemasa Miyoshi, and Misako Kachi, LETKF-based Ocean Research Analysis (LORA), The 5th R-CCS International Symposium, Kobe
– Other
Otsuka, S., T. Awazu, C. A. Welzbacher, R. Potthast, and T. Miyoshi, 2023: Assimilating precipitation features based on the fractions skill score: an idealized study with an intermediate AGCM. in “Numerical Weather Prediction: East Asian Perspectives”, edited by Park, S. K., Springer Nature, in press.
Usage of JSS
Computational Information
- Process Parallelization Methods: MPI
- Thread Parallelization Methods: OpenMP
- Number of Processes: 5 – 400
- Elapsed Time per Case: 12 Hour(s)
JSS3 Resources Used
Fraction of Usage in Total Resources*1(%): 2.99
Details
Please refer to System Configuration of JSS3 for the system configuration and major specifications of JSS3.
System Name | CPU Resources Used(Core x Hours) | Fraction of Usage*2(%) |
---|---|---|
TOKI-SORA | 81682519.97 | 3.56 |
TOKI-ST | 0.00 | 0.00 |
TOKI-GP | 0.00 | 0.00 |
TOKI-XM | 0.00 | 0.00 |
TOKI-LM | 0.00 | 0.00 |
TOKI-TST | 0.00 | 0.00 |
TOKI-TGP | 0.00 | 0.00 |
TOKI-TLM | 0.00 | 0.00 |
File System Name | Storage Assigned(GiB) | Fraction of Usage*2(%) |
---|---|---|
/home | 2113.33 | 1.91 |
/data and /data2 | 399153.33 | 3.08 |
/ssd | 1033.33 | 0.14 |
Archiver Name | Storage Used(TiB) | Fraction of Usage*2(%) |
---|---|---|
J-SPACE | 31.16 | 0.14 |
*1: Fraction of Usage in Total Resources: Weighted average of three resource types (Computing, File System, and Archiver).
*2: Fraction of Usage:Percentage of usage relative to each resource used in one year.
ISV Software Licenses Used
ISV Software Licenses Used(Hours) | Fraction of Usage*2(%) | |
---|---|---|
ISV Software Licenses(Total) | 0.00 | 0.00 |
*2: Fraction of Usage:Percentage of usage relative to each resource used in one year.
JAXA Supercomputer System Annual Report February 2022-January 2023